Explainable AI Examples In Real Life
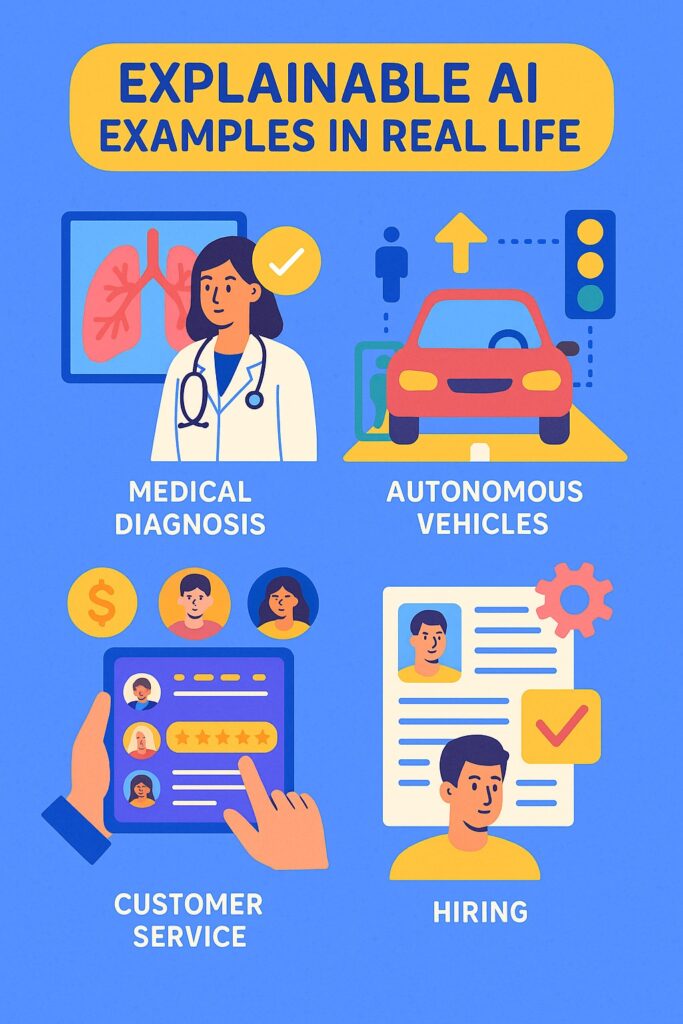
Artificial Intelligence (AI) continues to revolutionize industries, but its “black box” nature has raised concerns. That’s where Explainable AI (XAI) comes in. Explainable AI refers to systems that provide human-understandable insights into how decisions are made. In today’s real-world applications, XAI isn’t just a theoretical concept—it’s becoming essential for transparency, trust, and accountability. This article explores real-life examples where XAI is making a tangible difference.
Healthcare: Transparent Medical Diagnoses
One of the most impactful uses of explainable AI is in the healthcare sector. Traditional AI models can detect diseases like cancer or pneumonia from medical images, but without explanation, doctors may hesitate to trust the outcomes. Explainable AI addresses this concern by revealing how and why the diagnosis was made.
For example, in radiology, an XAI system might highlight the specific region of an X-ray that led it to suspect a lung abnormality. Doctors can then confirm or challenge the decision, combining clinical expertise with AI recommendations. This collaborative approach ensures greater accuracy, patient safety, and informed treatment decisions.
Another practical use is in predicting patient deterioration. AI models analyze medical histories, lab results, and vital signs to anticipate risks like sepsis. With explainable outputs, clinicians can understand which factors—such as low blood pressure or high heart rate—triggered the alert.
Autonomous Vehicles: Safer Navigation Decisions
Self-driving cars depend on AI to make split-second decisions. However, for these vehicles to gain public trust, their reasoning must be explainable.
Let’s say an autonomous vehicle chooses to slow down unexpectedly. An explainable AI system can clarify that it detected a pedestrian about to step onto the crosswalk. The system may further show that camera input, radar, and historical traffic patterns supported the decision.
This type of transparency is especially useful during accident investigations. Engineers and regulators can review AI decision trails to understand what happened and why. It also helps improve future system designs by identifying flaws or areas needing adjustment.
Financial Services: Fair Loan Approvals
Financial institutions are using AI for credit scoring, fraud detection, and loan approvals. However, these decisions carry serious consequences, and any appearance of bias can lead to regulatory scrutiny.
Explainable AI allows lenders to offer justifications for decisions. For instance, if a loan application is rejected, the XAI model can explain it was due to insufficient income, lack of credit history, or high existing debt. This transparency is crucial in complying with fair lending laws and preventing discrimination.
In fraud detection, explainable systems can point out unusual transaction patterns or behavior anomalies that triggered an alert. Investigators can quickly determine whether the activity is truly suspicious or a false positive.
Human Resources: Ethical Hiring Decisions
Recruitment and hiring increasingly involve AI systems to screen resumes and rank candidates. But concerns around bias have made explainability vital in this field.
If an AI tool recommends one applicant over another, HR professionals must understand the basis. Was it due to job experience, relevant skills, or education? XAI can break down the factors influencing a decision, helping recruiters ensure fair practices and justify choices if challenged.
For internal promotions or performance evaluations, explainable models can support transparency and mitigate workplace disputes. Employees are more likely to accept decisions when they understand the criteria used.
Customer Service: Personalized and Justified Responses
Many businesses now use AI-powered chatbots and recommendation engines to enhance customer service. Explainable AI plays a key role in ensuring these systems respond appropriately and fairly.
Imagine a chatbot denying a customer’s request for a refund. With XAI, the system can clarify that the item falls outside the return window or doesn’t meet policy criteria. This helps reduce confusion and enhances the customer’s trust in the process.
Similarly, when an AI suggests products or services, an explanation such as “based on your recent purchases” or “others with similar interests also bought this” makes the interaction more transparent and engaging.
Legal and Judicial Systems: Transparent Risk Assessments
In criminal justice, AI is being used for risk assessment—such as evaluating the likelihood of a defendant reoffending. These decisions can influence bail, parole, and sentencing outcomes.
Explainable AI is essential in this context to ensure fairness. Judges and lawyers need to see the data inputs and reasoning behind risk scores. Was the result influenced by past offenses, age, employment history, or another factor? Without clarity, such systems could reinforce existing biases or face legal challenges.
By providing human-understandable justifications, XAI enhances accountability and helps ensure that justice is served impartially.
Manufacturing: Predictive Maintenance and Quality Control
Factories use AI to predict equipment failures before they happen. Explainable AI helps technicians understand the warning signs and root causes.
For example, an XAI model might flag a motor for inspection and highlight data like increasing vibration levels or overheating trends. Maintenance teams can take preventive action and avoid costly downtime.
In quality control, explainable AI identifies defects during product inspection and explains what triggered the detection. This could be a subtle change in color, shape, or weight. Human inspectors can verify the issue and fix underlying problems in the production line.
Marketing and Advertising: Understanding Consumer Behavior
Marketing professionals use AI to analyze consumer behavior, optimize ad campaigns, and personalize experiences. Explainable AI enables them to interpret why certain strategies work.
If an AI platform recommends showing a particular ad to a user, the explanation might be that the user engaged with similar content or shares demographic traits with previous converters. This helps marketers adjust messaging, refine targeting, and increase campaign efficiency.
Furthermore, explainable insights reduce over-reliance on automated decisions. Marketers can assess whether models are focusing on the right metrics or unintentionally reinforcing stereotypes.
Agriculture: Smarter Crop Management
AI in agriculture helps monitor crop health, predict yields, and optimize irrigation. Explainable AI offers farmers actionable insights.
For instance, a model might advise reducing water in a specific section of a field. The system can explain that soil moisture readings and weather forecasts indicate over-irrigation. This empowers farmers to make informed decisions, conserve resources, and boost sustainability.
In pest detection, explainable models show which visual features—such as leaf discoloration or hole patterns—suggest pest activity, allowing timely intervention.
Energy Sector: Efficient Resource Management
Energy providers use AI to forecast demand, detect anomalies, and manage grids. Explainable AI supports safer and more efficient operations.
If an energy spike is predicted, the model can show that it’s based on temperature forecasts, previous consumption patterns, and regional data. This helps utility companies prepare and avoid blackouts.
In renewable energy, explainable AI is used to forecast solar or wind power generation. Operators can understand the variables at play—like cloud cover or wind speed—which enables better planning and storage strategies.
Conclusion: The Importance of Explainable AI Today
Explainable AI is no longer optional—it’s essential for ethical, transparent, and effective deployment of AI systems in real life. Whether in healthcare, finance, transportation, or beyond, explainability builds trust between humans and machines.
By integrating models that not only perform but also explain, organizations can ensure compliance, foster accountability, and improve user acceptance. As AI continues to shape the future, explainable systems will serve as the bridge between technical complexity and human understanding.
Key Takeaways:
-
Explainable AI (XAI) provides transparency into how AI systems make decisions, increasing trust and accountability across industries.
-
In healthcare, XAI helps doctors understand AI-driven diagnoses by showing relevant data and image highlights, improving patient outcomes.
-
Autonomous vehicles use XAI to explain decisions like stopping or changing lanes, which aids in safety reviews and public trust.
-
Financial services benefit from XAI by providing clear reasons for loan approvals or fraud alerts, ensuring compliance and fairness.
-
In human resources, XAI enables ethical hiring by revealing the factors behind candidate evaluations and selections.
-
Customer service chatbots and recommendation engines use XAI to justify decisions or suggestions, enhancing user experience and reducing complaints.
-
Legal systems leverage XAI to make transparent risk assessments in bail, parole, or sentencing, minimizing bias in judicial decisions.
-
Manufacturing operations use XAI for predictive maintenance and quality control by pinpointing exact issues in real time.
-
Marketing campaigns become more effective with XAI by explaining consumer behavior and the rationale behind ad targeting.
-
In agriculture, XAI assists farmers with irrigation, pest detection, and crop management by interpreting environmental and sensor data.
-
Energy sectors apply XAI to optimize power distribution, predict usage surges, and manage renewable energy resources efficiently.
-
Explainable AI is vital for ethical, reliable, and human-centered AI, empowering industries to make better decisions backed by clear reasoning.
References
https://en.wikipedia.org/wiki/Explainable_artificial_intelligence
Links License – https://en.wikipedia.org/wiki/Wikipedia:Text_of_the_Creative_Commons_Attribution-ShareAlike_4.0_International_License
Dear Friends, welcome you to click on link below for more tech articles. Have fun reading them. Thanks For Your Support.
https://techsavvo.com/category/blog/